What is Explainable AI (XAI) and Why You Should Care
As technology continues to evolve and business executives look for the best way to keep their operations running smoothly, it’s important that they stay informed on the latest trends in data-driven technologies.
Chief among them is explainable AI (XAI), which has the potential to revolutionize how companies process and interpret large amounts of data in order to drive insightful decisions. We are living in a data-driven world and AI is at the helm of it. But with great power comes great responsibility, which is why XAI is a must-have for any business looking to succeed moving forward.
What is Explainable AI?
Artificial intelligence is becoming more complex and increasingly implemented across society, which makes explainability even more crucial.
IBM provides a simple but effective definition for XAI:
”Explainable artificial intelligence (XAI) is a set of processes and methods that allows human users to comprehend and trust the results and output created by machine learning algorithms”
XAI helps describe an AI model, its expected impact and potential biases. All of this leads to better model accuracy, fairness, transparency and outcomes when AI is used for data-driven decision making.
Explainability is critical as AI algorithms take control of more applications and sectors, which brings along the risk of bias, faulty algorithms, and various other issues. By ensuring transparency for your company through explainability, you can truly leverage the power of AI.
Explainable AI is not just one single tool but rather a set of tools and frameworks that help you, your company and the public understand and interpret predictions made by machine learning models.
Solving the “Black Box” Effect with XAI
In contrast to explainable AI models, many of today’s AI systems are so advanced that humans must carry out a calculation process to retrace how the algorithm arrived at its result. This process leads to the “black box” effect where it is impossible to understand how the model came to its decision. These “unexplainable AI” models are usually developed directly from the data, and nobody knows what’s taking place inside them.
The effectiveness of AI systems is limited by the machine’s inability to explain its decisions and actions to us humans, and as these machines become more intelligent and autonomous, this becomes even more difficult.
XAI attempts to solve this problem in a few different ways. For one, it helps produce more explainable models while still maintaining a high level of learning performance. It also enables users to understand, trust, and manage new systems.
Machine learning is quickly becoming an integral part of our everyday lives and in order to trust these systems, we need to know exactly how and why they are making their decisions. Researchers are developing new approaches that will provide us with transparent models along with intuitive user interfaces for understanding them. By combining machine-learning techniques and human-computer interface technology, we can improve the safety of machine learning and our own problem solving skills in the long run. After all, by having insight into how machines learn, perhaps humans can gain deeper insights into our own thought processes.
XAI is one of the various approaches and technologies that make up “third-wave AI systems,” which are machines that understand the content and environment they are operating in. These systems can eventually characterize real world phenomena by building underlying explanatory models.
By understanding the logic behind the predictions of a machine learning algorithm, the black box effect goes away. This helps your company’s data scientists and ML engineers explain the reasoning behind the predictions, and it helps the overall organization trust and adopt AI technologies.
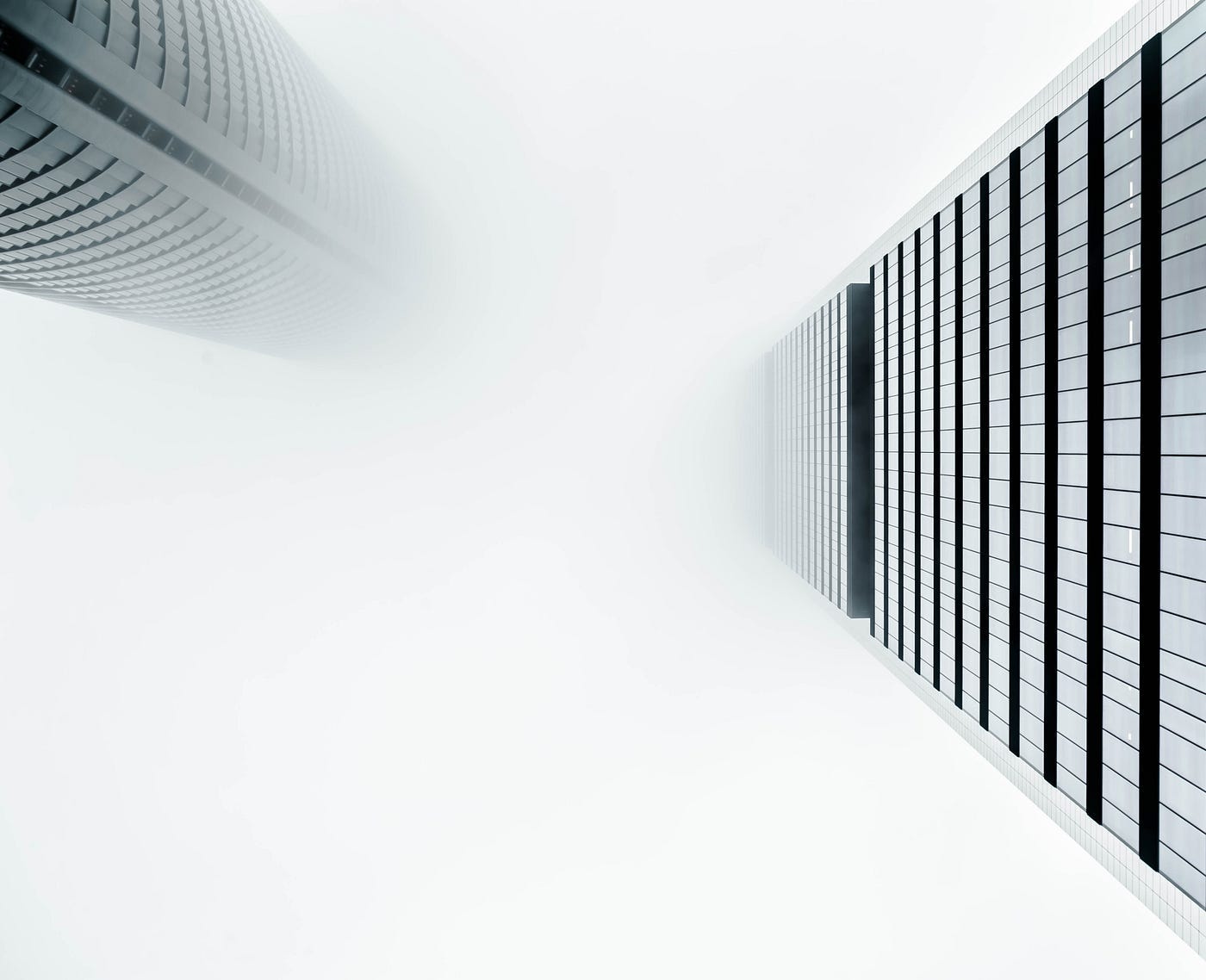
The Ethical Importance of Explainable AI
Understanding the AI decision-making process is critical for any organization, as blindly trusting them could easily lead to unforeseen problems. Explainable AI can provide a valuable tool to assist humans in understanding, explaining and evaluating machine learning algorithms, deep learning networks, and neural networks — providing a way to monitor their accuracy and explain why certain decisions were made. With XAI, it can be much easier to administer accountability with regards to AI decision-making, ultimately resulting in more ethical technology solutions.
Bias in AI has been a notorious issue for a long time, based on race, gender, location and more. For example, the COMPAS tool, which was created as a decision support tool to asses the sentencing and parole of convicts, was found to exhibit racial bias. Another example is software used by banks to determine whether or not someone will pay back credit-card debt, which was also found to exhibit bias.
If algorithms like these biased ones offered better explanations behind their reasoning, some of the issues could be contained quickly. The bias would be easier to detect and the algorithms could be fine-tuned to provide better and fairer outputs.
For businesses leveraging AI models in production environments, explainable AI is essential for monitoring, managing and creating trust among end users. Ultimately, XAI can help promote auditability and make sure that compliance, legal and security risks are mitigated while still harnessing the benefits of AI. Explainable AI should be used as a critical tool to ensure stability of production workflows through continuous measurement of its business impact.
XAI is also an important component of responsible AI, providing a methodology for integrating AI into your organization in an ethical and accountable way. It helps develop transparent models with explainability, fairness and trustworthiness. This encourages your company to infuse ethical considerations into the design, implementation and maintenance of AI applications and systems.
Adopting XAI is the key for successful implementation of responsible AI and allows your organization to build lasting trust with users. It ensures that applications are built on a foundation of safety and security.
The Benefits of Explainable AI
There are many ways explainable AI can benefit your organization as it creates the conditions needed for professionals to leverage the most value from AI systems. With XAI, technologists can more efficiently monitor, maintain and improve AI systems. At the same time, business professionals will have a higher level of trust in AI outputs and legal professionals will have a better view of whether technology and associated workflows comply with regulations and customer expectations.
Besides these benefits, explainable AI also helps:
Increase Productivity: The advent of explainable AI solutions offers MLOps teams a much-needed way to verify the accuracy and reliability of their AI systems. By taking into consideration specific features which drive model output, technical professionals are able to discern if certain patterns reflect general trends that can be applied going forward — or whether they’re merely atypical correlations from past data points.
Build Trust: For any AI model to be successful, it must inspire trust in its users. Explaining the basis for recommended actions is key here — when customers and regulators can understand why something has been suggested, they will develop a stronger faith in the outputs of this technology. Even state-of-the-art algorithms won’t be embraced without understanding their inner workings — sales teams are more likely to listen if an AI’s suggestions make sense rather than seeming like the random output from a ‘black box.’
Business Value: By arming technical and business teams with the insights needed to understand how AI works, you can ensure that your applications are built to maximize value. By understanding both sides of the equation — its technology capabilities as well as intended objectives — organizations stand a much greater chance of capitalizing on their full potential.
New Business Interventions: Your company can benefit from more than just the predictions generated by AI models. By delving deeper into these systems and learning why a certain prediction was made, your organization can uncover opportunities for powerful interventions within the business that would otherwise remain unknown. For instance, utilizing explanations of customer churn likelihood in various segments to recognize where your business should focus resources provides greater value than simply understanding what’s likely to happen.
Mitigating Risks: Organizations turn to explainability so they can safely navigate around potential risks posed by AI systems. Drafting clear ethical guidelines helps guard against any accidental missteps that may draw unwanted attention from the media, public and regulators alike. Legal teams use this valuable insight alongside their intended business purpose to ensure compliance with all laws and regulations as well as adherence to company policies and values
Use Cases for XAI
There are many use cases for XAI, and it should be considered by every AI-driven organization no matter the industry. It is critical to creating a more trustworthy and transparent relationship between your business, its employees and the public.
Fraud Detection
XAI is an incredibly useful tool when it comes to fraud detection. Not only does it make a prediction on what may be fraudulent, but it offers a human-readable explanation as to why it thinks a transaction is fraudulent — something traditional machine learning simply cannot do.
This means users can easily understand why their transactions were deemed suspicious, rather than battling the frustration of seemingly random occurrences. Additionally, in cases of false predictions, explanations would help to pinpoint and resolve system errors quickly and effectively.
Online Recommendations
Explaining the “why” of a recommendation can be highly beneficial. Through XAI, customers have much higher chances of getting relevant and personalized recommendations tailored to their needs.
This increases customer satisfaction, with an improved overall experience transforming into loyal customers who return again and again. Not only that, but Implementing XAI can increase your target market size, as well as sales and conversion rates per individual customer.
Credit and Loan Decision Making
AI can revolutionize the way financial services are granted to individuals and businesses. By implementing explainable AI, individuals and businesses now have greater transparency around why they may be denied or accepted for certain services.
XAI also makes processes more auditable, with traceability into each decision-making process. This helps ensure that the decisions are legal, ethical and compliant — something which is of utmost importance in any sector. The addition of XAI can help your company have confidence that its use of AI creates tangible value while being respectful of laws and regulations.
Manufacturing
Utilizing XAI while monitoring machinery on an assembly line can be highly beneficial. This technology can use machine-to-machine communication to help detect issues before they even occur, which gives the machines themselves greater situational awareness.
It can also be used to understand what adjustments need to be made over time to ensure that the assembly line remains functioning in an optimal manner. Ultimately, XAI is a key asset for manufacturers as it provides greater understanding and control of automated systems, thus ensuring efficient yields and profits.
Autonomous Vehicles
With the rise of autonomous vehicles, enhanced safety measures have become even more paramount. AI algorithms utilized in these vehicles require explainability techniques to ensure decisions are made with responsibility and accountability.
XAI comes into play here by gaining situational awareness in cases of unexpected events, helping reduce crashes and promote safer systems on the whole. This is why XAI for automotive industry applications is so valuable; its implementations enable responsible technology operation, increased safety regulation, and increase trust in self-driven automobiles.
As we continue to progress further in the development of autonomous vehicles, explainable AI will become a standard part of safety protocol.
Military
XAI offers an invaluable approach for military training applications, providing an often-lacking layer of transparency. One of its key benefits is that it can explain why an artificial intelligence system opted to recognize an object incorrectly or not fire on a target.
Not only does this feature offer practical benefits, such as allowing AI systems to be held to higher ethical standards, but it gives trainers greater control and the ability to prevent future ethical dilemmas — a crucial priority. With XAI, developers can ensure their autonomous systems are making decisions in compliance with mission objectives and applicable laws.
(To learn more about how AI is used for military applications, check out my following articles: “AI and Warfare,” “The Augmented Soldier,” and “Modern Information Wars.”)
These are just a few of the many potential applications of explainable AI, but the centrality of XAI is such that it should be thought of as a central element of any AI project going forward.
XAI is an Absolute Must for Today’s AI-Driven Businesses
Explaining AI is becoming increasingly important as more businesses implement machine learning algorithms into their operations. With XAI, business executives have the ability to gain insight into how these algorithms are making decisions and use this information responsibly to make informed decisions about their operations moving forward.
Explainable AI is an indispensable tool for businesses looking to stay ahead of the curve when it comes to handling large amounts of data efficiently and accurately while also building customer trust through transparency. By understanding how machine learning algorithms make decisions through explainability tools, you can ensure the business is making informed decisions that accurately reflect customer needs while also increasing efficiency and accuracy throughout its operations.
For any business eager to stay competitive in this ever-evolving landscape, XAI should be a top priority when considering current trends designed for improving operations today — and tomorrow!
>>> Keep a lookout for my follow-up piece, which will cover the technical aspects of explainable AI.
>>> Follow MVYL on Twitter, LinkedIn and Instagram for more AI-related content.